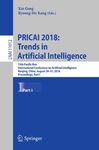
PRICAI 2018: Trends in Artificial Intelligence
Role
Editors: Xin Geng, Byeong-Ho Kan
Contributing authors: Yujia Zhang, Jun Wu, Haishuai Wang
Files
Document Type
Conference Proceeding
Description/Summary
Haishuai Wang (with Yujia Zhang, Jun Wu) is a contributing author, "Binary Collaborative Filtering Ensemble."
Book Introduction:
This two-volume set, LNAI 11012 and 11013, constitutes the thoroughly refereed proceedings of the 15th Pacific Rim Conference on Artificial Intelligence, PRICAI 2018, held in Nanjing, China, in August 2018. The 82 full papers and 58 short papers presented in these volumes were carefully reviewed and selected from 382 submissions. PRICAI covers a wide range of topics such as AI theories, technologies and their applications in the areas of social and economic importance for countries in the Pacific Rim.
Paper abstract:
We address the efficiency problem of Collaborative Filtering (CF) in the context of large user and item spaces. A promising solution is to hash users and items with binary codes, and then make recommendation in a Hamming space. However, existing CF hashing methods mainly concentrate on modeling the user-item affinity, yet ignore the user-user and item-item affinities. Such manner results in a large encoding loss and deteriorates the recommendation accuracy subsequently. Towards this end, we propose a Binary Collaborative Filtering Ensemble (BCFE) framework which ensembles three popularly used CF methods to preserve the user-item, user-user and item-item affinities in the Hamming space simultaneously. In order to avoid a time-consuming computation of the user-user and item-item affinity matrices, an anchor approximation solution is employed by BCFE through subspace clustering. Specifically, we devise a Discretization-like Bit-wise Gradient Descend (DBGD) optimization algorithm that incorporates the binary quantization into the learning stage and updates binary codes in a bit-by-bit way. Such a discretization-like algorithm can yield more high-quality binary codes comparing with the popular “two-stage” CF hashing schemes, and is much simpler than the rigorous discrete optimization. Extensive experiments on three real-world datasets show that our BCFE approach significantly outperforms state-of-the-art CF hashing techniques.
ISBN
9783319973036
Publication Date
2018
Publication Information
Zhang Y., Wu J., Wang H. (2018) Binary Collaborative Filtering Ensemble. In: Geng X., Kang BH. (eds) PRICAI 2018: Trends in Artificial Intelligence. PRICAI 2018. Lecture Notes in Computer Science, vol 11012. Springer, Cham. https://doi.org/10.1007/978-3-319-97304-3_76
Recommended Citation
Geng, Xin; Kan, Byeong-Ho; Zhang, Yujia; Wu, Jun; and Wang, Haishuai, "PRICAI 2018: Trends in Artificial Intelligence" (2018). Engineering Faculty Book Gallery. 28.
https://digitalcommons.fairfield.edu/engineering-books/28
Comments
© Springer Nature Switzerland AG 2018